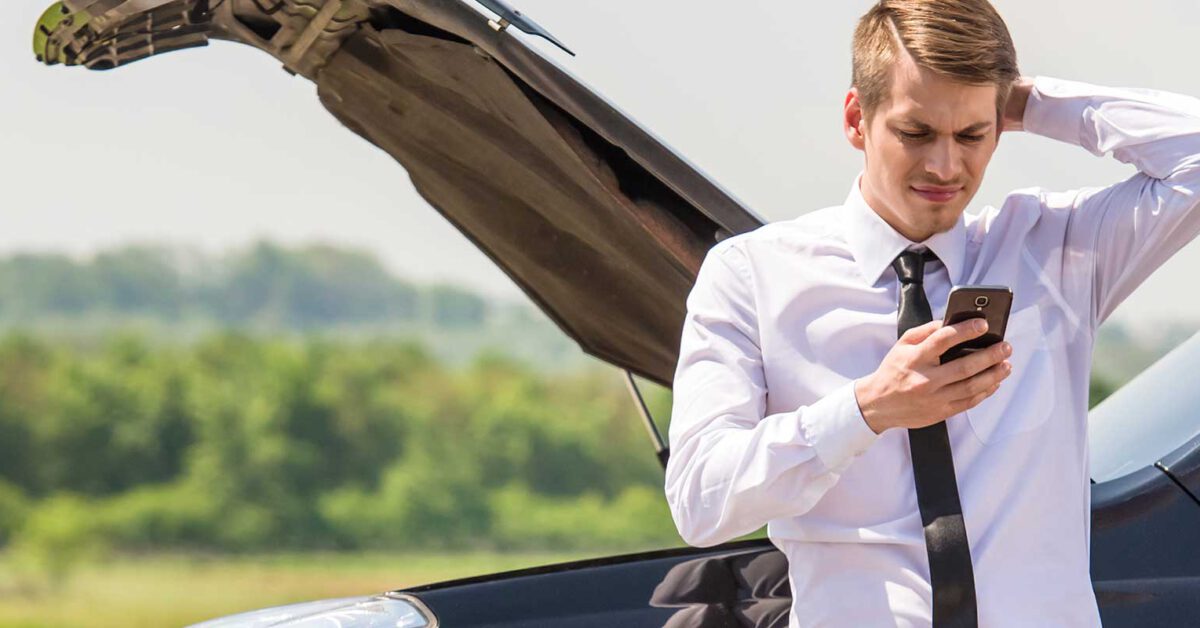
Yesterday, while in the middle of a work frenzy, my WiFi went down. With one failed router, my computer went from a high-tech hub with team wide communication applications and the world’s research at my fingertips, to a sad Mac with nothing but a word processor and a blinking cursor. Now, in the grand scheme of things, my downtime was negligible. I was able to get the internet back on my side within an hour, and content continued to be created on into the evening. But, in industries all over the world, downtime happens, and it when it does, it can be very expensive. And in the case of manufacturing, it can be downright devastating.
Downtime can generally be defined as a period of time when something such as a network, factory, or piece of machinery is not in operation – specifically as a result of some sort of failure or malfunction. And if you’ve ever had one of these things break down at a critical phase of a project, then you know exactly how that downtime can impact your schedules, your brand reputation, and your overall profit potential.
What’s Your True Downtime Cost?
Speaking of profit potential, let’s talk about the actual cost of downtime. True downtime cost (TDC) includes all the business support costs dedicated to maintenance and repair time, added to the potential lost business opportunity costs. From tangible costs like lost capacity, lost production, direct labor and inventory, to the intangibles like responsiveness, stress, and reputation, some TDC can be difficult to earn back.
According to Arimo, a behavioral artificial intelligence (AI) platform, manufacturers have to deal with up to 800 hours of downtime annually, and, on average an automotive manufacturer’s TDC is $22,000 per minute. Do the math – that’s $1.3M per month!
So, what’s actually at the root of this TDC? In many cases, it’s the manufacturer’s reliance on a preventative maintenance model rather than a predictive maintenance model. While the two seem (and even sound) similar, preventative maintenance is actually just maintenance based on fixed schedules like time, events, and meter readings, and it’s a known method in many manufacturing maintenance processes. But how does it differ from predictive maintenance?
Preventative vs. Predictive Maintenance
An example by Core Systems describes the difference best by comparing the methods of maintenance on both an economy car and a luxury car. The economy car’s manual suggests a preventative oil change after three months or 3,000 miles. This makes sense if you’ve driven 3,000 miles in three months. But, if you’ve only driven the car 1,000 miles, this is an example of how preventative maintenance, i.e. fixed planning, isn’t the most intelligent. With that luxury car, however, you would have a service sensor on your engine oil that sends an alert 500 miles before your engine fails, essentially predicting failure AND preventing it.
Now, think back to the Rolls Royce example from PPS: The company started by investing money in a data warehouse, not even knowing what to do with that data at the time, and, through time series and motion studies on active aircrafts, they shifted to predictive maintenance and began to fix parts between flights. Their messaging shifted from “you need an aircraft engine,” to “you need an aircraft engine that is functioning when you need it to function,” and they’re now positioned as a service organization focused on Engine Uptime and predictive maintenance.
Data, Data, Data
A common theme in organizations struggling to make this same change today? Data. According to Don Fitchett, founder of Business Industrial Network, predictive maintenance and analytics like TDC come from “analyzing all cost factors associated with downtime, and using this information for cost justification and day to day management decisions.” But in the case of many manufacturers, “this data is already being collected and simply needs to be consolidated and organized according to the TDC guidelines.”
But, when used correctly, data and predictive maintenance can be realized through the application of sophisticated machine learning techniques, and it’s now the new standard for reducing cost, risk and lost production in manufacturing. “With accurate predictive maintenance tools,” says Arimo, “manufacturers can choose to do maintenance only when needed, avoid costly or dangerous unplanned downtime, or schedule repair and maintenance personnel and resources more efficiently.”
So, while new product-based revenues may be declining, after-sales service margins are continuing to provide new revenue and profit opportunities. Today’s most successful companies are looking beyond the new product side of their businesses and shifting the way they view post-sales service, countering the dangerous costs of downtime with modern and predictive methods of product uptime.