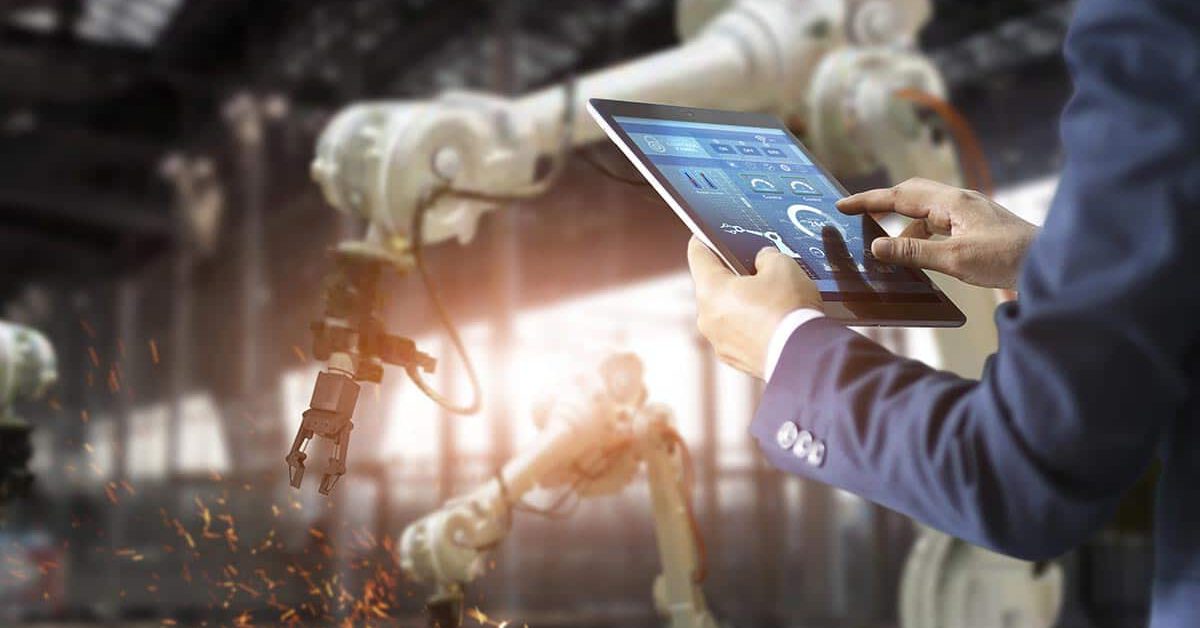
We’ve been talking a lot recently about using inventory and pricing tactics to navigate the post-pandemic economy. This week we want to shift focus and look at the more futuristic side of servitization. For OEMs and manufacturers making the move to selling products-as-a-service (PaaS), avoiding unplanned downtime becomes increasingly vital to protecting your bottom line. To maximize product uptime, you need to keep all your equipment running optimally; maintenance strategies must be adapted to incorporate predictive and proactive approaches in addition to the traditional reactive and preventive approaches. While you’re probably familiar with preventive maintenance, let’s understand how predictive maintenance is different. We’ve found there’s some discrepancy between how these two terms are used, so let’s start with some definitions.
The three P’s of maintenance: Preventive, Predictive, and Proactive
Preventive or scheduled maintenance refers to tasks that are done on a fixed schedule based on time or usage criteria, regardless of the equipment’s actual condition. Predictive maintenance on the other hand involves continuously monitoring an asset via sensor data, typically collected using telematics or IoT enabled devices, and using that data, often together with modern technologies like AI or machine learning, to predict an imminent failure before it happens. Manufacturers are also starting to talk about proactive or prescriptive maintenance, which is a more specialized form of predictive maintenance that looks at known failure models and prescribe proactive actions to optimize desired outcomes. Industry also refers to this as prescriptive maintenance.
The confusion between predictive and preventive maintenance often comes down from the use of underlying technology. In modern times, the time or usage intervals for Preventive maintenance are usually derived using some form of predictive modeling, such as mean time between failure (MTBF) curves. Irrespective of how the interval is derived, maintenance performed on a fixed schedule without continuous assessment of the underlying condition of the asset is still preventive maintenance. True predictive maintenance must be based on the specific condition of a machine or piece of equipment that’s indicating imminent failure, assessed with the help of continuous sensor data.
Service maturity models: balancing risk with reward
As manufacturers shift away from the traditional sales models towards servitization, predictive maintenance becomes increasingly important. OEMs often include an uptime guarantee when selling fixed price service packages, and the more revenue and margins those service packages account for, the more critical uptime of that underlying asset becomes. With a pure product-as-a-service (PaaS) business model, any downtime at all puts your revenue—and your business—at risk.
Where you are on the service maturity scale depends on your business model. Based on our research, we have found that OEMs that primarily serve customers through an independent dealer network are generally focused on preventive maintenance, while manufacturers that are further along the path to servitization are focusing on predictive maintenance. This includes large industrial equipment OEMs that predominantly sell or service their customers directly—for example, power generation equipment, transportation equipment, aerospace & defense, and so on. These industries tend to sell a more mature maintenance package with their equipment, which often includes SLAs guaranteeing availability or reliability.
While adopting a product-as-a-service model does increase the risks associated with downtime, it’s still a smart move for OEMs in the long run. Customers are demanding increased service offerings to simplify their own businesses, and the broader cultural shift driven by the gig economy and consumption of apps and services is also impacting manufacturing. Although riskier, PaaS models are also much more profitable than traditional sales with higher margins and steadier recurring revenue streams. This tremendous opportunity makes managing the risk well worth it. Now let’s look at some common challenges and how predictive and proactive maintenance can help you address them.
Unplanned downtime: Some facts
According to an ARC Advisory Group study from 2015, only about 20% of failures that contribute to downtime are strongly correlated with factors such as time or usage. And of those failures, companies only successfully avoid unplanned downtime about half the time because they lack necessary spare parts during the planned maintenance event.1 Despite this, most preventive maintenance programs define maintenance intervals based on time and usage metrics. Even industries with the most mature preventive maintenance programs, such as continuous process manufacturing industries, continue to lose a tremendous amount of money each year due to unplanned downtime resulting from asset failures.
The ARC study also found that the other 80% of failures occur at more random times, spread out over an asset’s lifetime.1 For example, internal engine components on a commercial truck fail randomly from a variety of reasons: extreme environmental and operating conditions, degraded lubricating oil, dirty filters etc. The only way to eliminate this type of symptom-based failure is to take a condition-based, proactive analytics approach leveraging modern technology such as machine learning. With the right solution, you can reduce asset downtime by up to 40%, realize a 30% reduction in maintenance costs, and improve your first-time fix rate by up to 30%. The payoff in terms of margins, revenue, customer satisfaction and loyalty is tremendous.
Keeping equipment running the old school way
The traditional PF (Performance-to-Failure) curve, which charts the progressive degradation and performance of an asset (Y axis) over time (X axis) is a great way to illustrate the differences in the various approaches to maintenance, and thus understand the benefits of predictive and proactive approaches. We know that before an asset fully fails, it generally exhibits symptoms which, if monitored and detected, will enable you to prevent the failure or at least reduce the downtime. The earlier you detect an imminent failure, the less costly it is to fix the problem. For example, if you identify a corrosion or leak in the oil cooler that’s degrading the lubricating oil, you can fix that—which is easier and less expensive than fixing a bearing wipe. Catching these “symptoms” sooner can also help prevent a catastrophic failure.
The oldest and most well-known way to identify failures ahead of time is manual inspection. However, this method leaves little time to react and oftentimes major damage is already done. A slightly more advanced option is condition monitoring, which essentially automates that human inspection. This involves monitoring an asset’s sensor data and applying domain- and design-specific thresholds on single parameters such as temperature, pressure, vibrations, and so on, to trigger an alert when it goes out of the expected range. This method is better than manual inspection, but still only leaves you with limited options as awareness comes very close to the point of failure. These approaches are also very hard to scale by defining accurate thresholds balancing competing factors of early warning and the false alarm rate, while accounting for the variation in environmental & operating characteristics, age, manufacturing processes & tolerances, and maintenance practices for these assets – each of which impact the normal behavior and performance. In other words, an abnormal and degraded performance for one asset maybe perfectly normal and acceptable for another asset.
Predicting problems sooner
The next step along the evolution of maintenance is anomaly detection, which is another form of condition-based maintenance that uses a multi-variate machine learning approach. This means using sensor data to look at multiple variables simultaneously and model a system’s normal behavior based on its past behavior. You can create individual models or a digital twin for each asset. By comparing the current behavior (as measured by sensor data in real time or close to it) to a historical healthy baseline, the model is able to detect and flag any abnormal behavior. A multi-variate model is able to detect small relative changes in data in any sensor before the change becomes significant enough for the human eye to identify it. When presented in an appropriate way with the right context, an expert can then analyze this behavior and determine the root cause. This approach enables earlier detection of a potential failure, giving you valuable time to diagnose and correct the issue at the least possible cost—for example, ordering parts or scheduling service before the asset fails.
An even more advanced condition-based approach is failure prediction, which involves creating machine learning models to look for precursor data patterns leading up to specific known failures. These patterns occur before the start of a failure and can only be identified using advanced algorithms. An example would be subtle changes in the combination of fuel flow + fuel pressure + engine efficiency that signifies a decrease in engine performance even before you would be able to see any impeller wear in the fuel pump or piston ring damage. By identifying the symptoms that lead to the start of a failure with known consequences, you can recommend operational changes to delay or even prevent it. On the flip side, if any downtime resulting from an early intervention is deemed to be more expensive than the probability of the known consequence, you can make an informed decision to not take any action (i.e. Use a Run-to-failure strategy), achieving the optimum desired outcome. This is what prescriptive maintenance is all about.
Both anomaly detection and failure prediction help you identify potential failures earlier to reduce unplanned downtime, extend the overall life of equipment, and reduce maintenance costs. The primary difference is that anomaly detection identifies potential failures with unknown consequences, whereas failure prediction looks for specific patterns in the data that lead to the start of a specific failure with a known consequence.
Next week we’ll look at challenges to overcome and practical steps to take when adopting a condition-based maintenance program. In the meantime, if you’re interested in putting your IoT sensor data to work to reduce product downtime and support your move to a servitization model, check out Syncron Uptime™.
[1] ARC Advisory Group, 2015: Asset Reliability, Software & Service Global Market Research Study